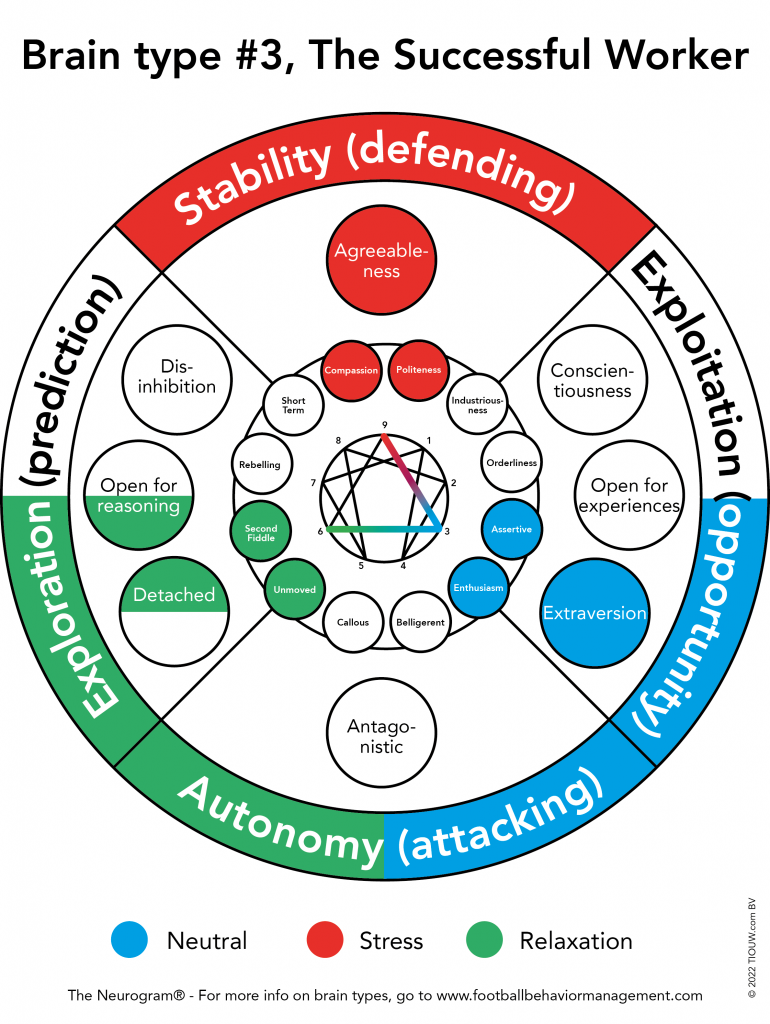
Exploitation
In football terms, “exploitation” typically refers to a player’s or a team’s ability to take advantage of the weaknesses or mistakes of the opposing team. This can be done in various ways, such as exploiting gaps in the defense, taking advantage of a goalkeeper’s poor positioning, or capitalizing on a defender’s lack of speed or skill.
Ruud Gullit was an exceptional footballer who was known for his versatility, technical skills, and physical prowess. He played in various positions throughout his career, including as a midfielder, forward, and sweeper. This adaptability allowed him to exploit mismatches against opponents who were less skilled or less physically gifted.
Gullit’s understanding of the game, combined with his technical skills, allowed him to create scoring opportunities for himself and his teammates. He could exploit gaps in the opposing team’s defense and could use his strength and skill to win physical battles against defenders. His ability to read the game also allowed him to anticipate where the ball was going to be and to position himself accordingly, giving him a further advantage over his opponents.
Autonomy
In a football context, “autonomy” could refer to a player’s ability to make independent decisions during a match, to control the game, and to take actions without needing explicit instructions or assistance from their teammates or coaches.
Ruud Gullit was known for his high football IQ, technical skills, and leadership abilities. These traits gave him a high degree of autonomy on the pitch.
As a player, Gullit had the ability to read the game and make decisions quickly. He could adjust his play style based on the situation, such as knowing when to hold onto the ball, when to pass, and when to attempt a shot on goal. He was also known for his ability to take on and beat defenders one-on-one, which is a skill that requires a high level of autonomy.
As a captain and a leader, Gullit often took charge on the pitch. He could direct his teammates, organize the team’s formations and strategies, and influence the flow of the game. His strong leadership skills are another indicator of his high level of autonomy.
Extraversion
“Extraversion” is a trait often associated with outgoing, sociable, and energetic individuals. Extraverts often enjoy being around people, are full of energy, and often quite active. They are likely to enjoy group activities and community participation.
Ruud Gullit, as a footballer and later as a manager, was often seen as an outgoing and charismatic figure. His public persona has often been characterized by a high level of energy and enthusiasm, both on and off the pitch. His ability to communicate effectively with teammates, lead as a captain, and later manage teams also indicates a high level of extraversion. He has also been involved in media work, further demonstrating his comfort in social and public situations.
Assertive
“Assertiveness” in a football context typically refers to a player’s ability to express their ideas and opinions clearly, confidently, and directly. It can also refer to their tendency to take charge in situations, to make their presence felt on the pitch, and to influence the actions of their teammates and opponents.
Ruud Gullit was known for his assertiveness both as a player and as a manager. As a player, Gullit was a commanding presence on the pitch. He was not afraid to take on opponents, to demand the ball from his teammates, or to take shots on goal. He was known for his ability to influence matches, whether by scoring goals himself, setting up his teammates, or disrupting the play of his opponents.
As a captain, Gullit was a vocal and influential leader. He would organize his teammates, direct play, and communicate clearly and effectively with both his team and the match officials. As a manager, Gullit was similarly assertive, making strategic decisions, managing his players, and dealing with the press.
Enthusiasm
Enthusiasm in the context of football generally refers to a player’s passion, energy, and dedication for the game. Enthusiastic players are often seen as being highly motivated, eager to participate, and having a positive attitude both on and off the pitch.
Ruud Gullit, throughout his career as a player and then as a manager, consistently demonstrated high levels of enthusiasm for football. Here are some possible reasons why Gullit might score high on enthusiasm:
- Passion for the Game: Gullit always showed a deep love and passion for football, which is a key component of enthusiasm. He was known for his dedication to improving his skills and understanding of the game.
- Energetic Play: Gullit was known for his energetic and dynamic style of play. He often took charge in matches, showing a keen desire to influence the game.
- Positive Attitude: Gullit often displayed a positive attitude, both in success and in adversity. This is a trait commonly associated with enthusiasm.
- Leadership: As a captain and a manager, Gullit showed enthusiasm in leading his teams. He was known for his ability to inspire and motivate his teammates.
- Post-playing Career: Even after retiring as a player, Gullit’s enthusiasm for football remained evident. He transitioned into management and punditry, roles that allowed him to stay involved with the sport he loves.